Data management
Build a solid foundation for growth and innovation
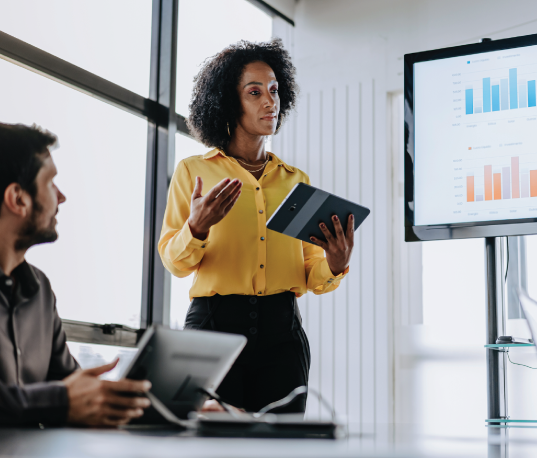
Businesses accumulate vast amounts of data in different formats from various systems - resulting in issues with access and accuracy. This fragmented landscape stands in the way of your ability to harness valuable data to inform decisions and meet the evolving demands of your organization.
EXL specializes in designing and implementing modern, scalable data management services and platforms based on your unique business requirements and strategic vision. We help you organize, integrate, clean, and secure your data - so you can unlock its true value and drive sustainable growth.
Build a solid foundation for growth and innovation
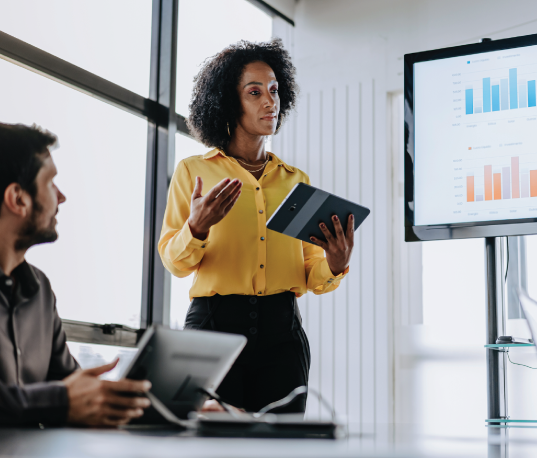
Our data management consulting
Are you confident that your data reflects your reality? We can help you make sense of vast amounts of information in complex systems to inform better decisions and drive measurable, long-term business results.
How does a modern data management strategy enhance performance?
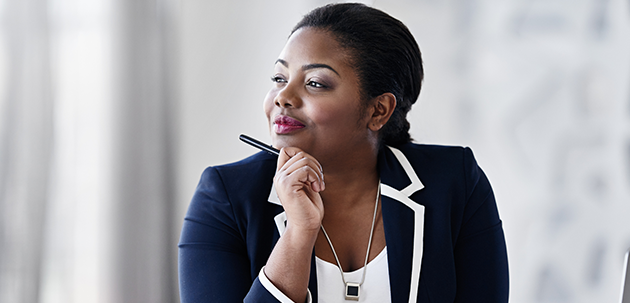
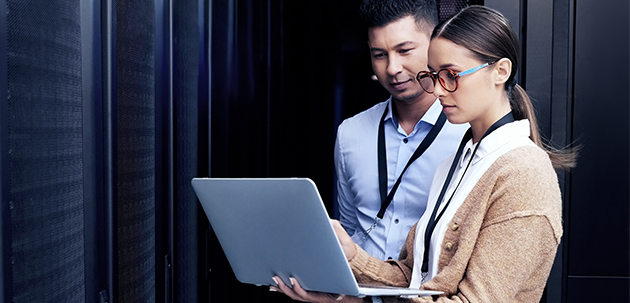
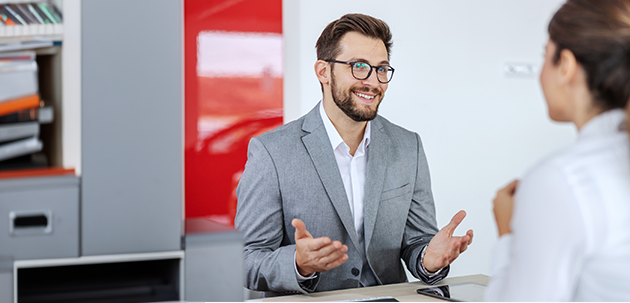
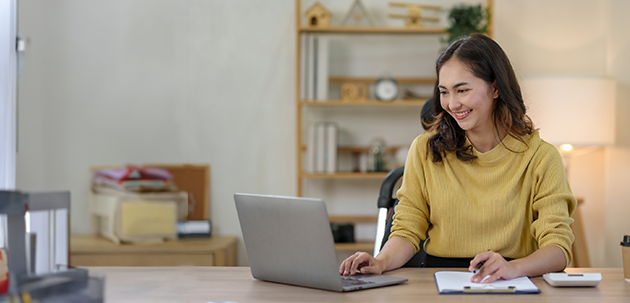