Transforming healthcare with data-led, AI-driven solutions
At EXL, we empower healthcare leaders to overcome the most complex challenges in today's evolving landscape. From managing rising costs and operational inefficiencies to navigating complex regulations and improving patient outcomes, our data-driven solutions are designed to solve real-world problems.
By combining a human-in-the-loop approach with deep domain expertise and cutting-edge proprietary technologies, we drive meaningful and lasting healthcare transformation.
EXL Impact
Powering the success of healthcare payers, providers, PBMs, and life science organizations.
6,000+
Healthcare professionals including 1,900+ clinical resources
300+
Coding and claims experts
260+ million
Unique life touch points
550+
Data scientists
Who we serve
Together, we work with clients across the healthcare ecosystem to meet their current and dynamic business challenges.
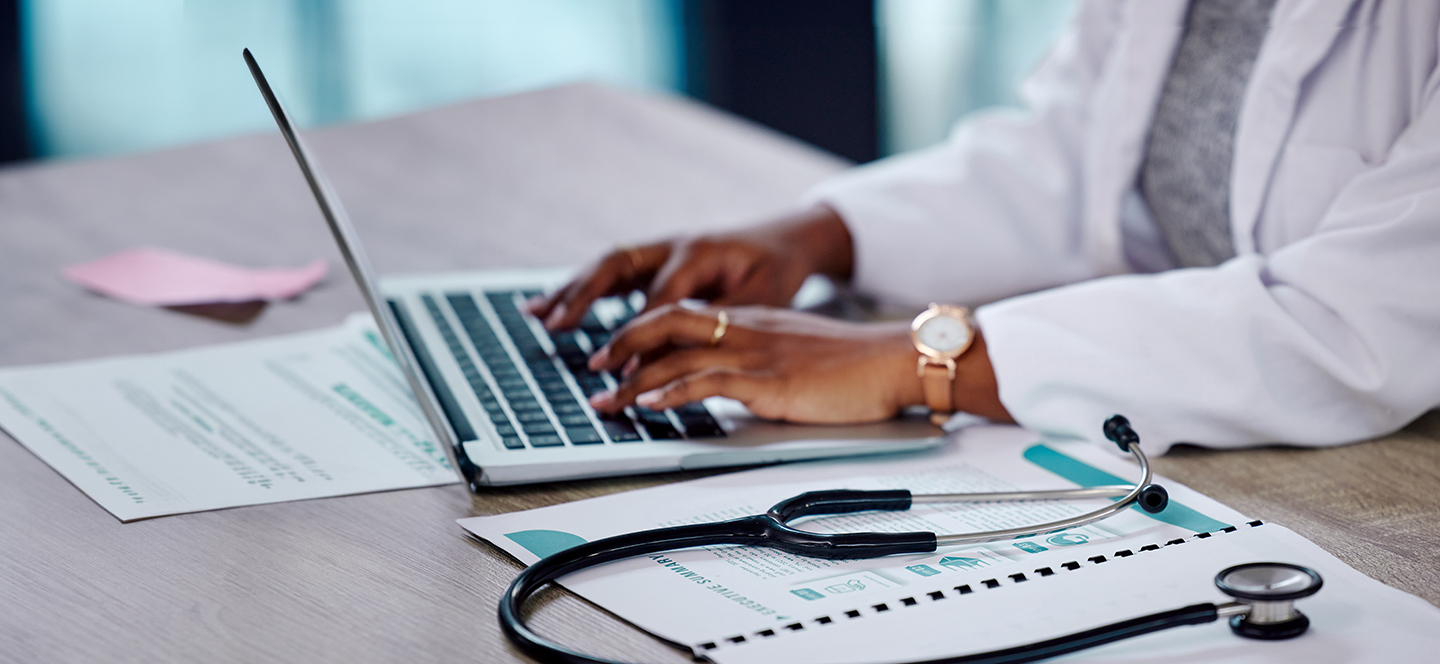
Payers
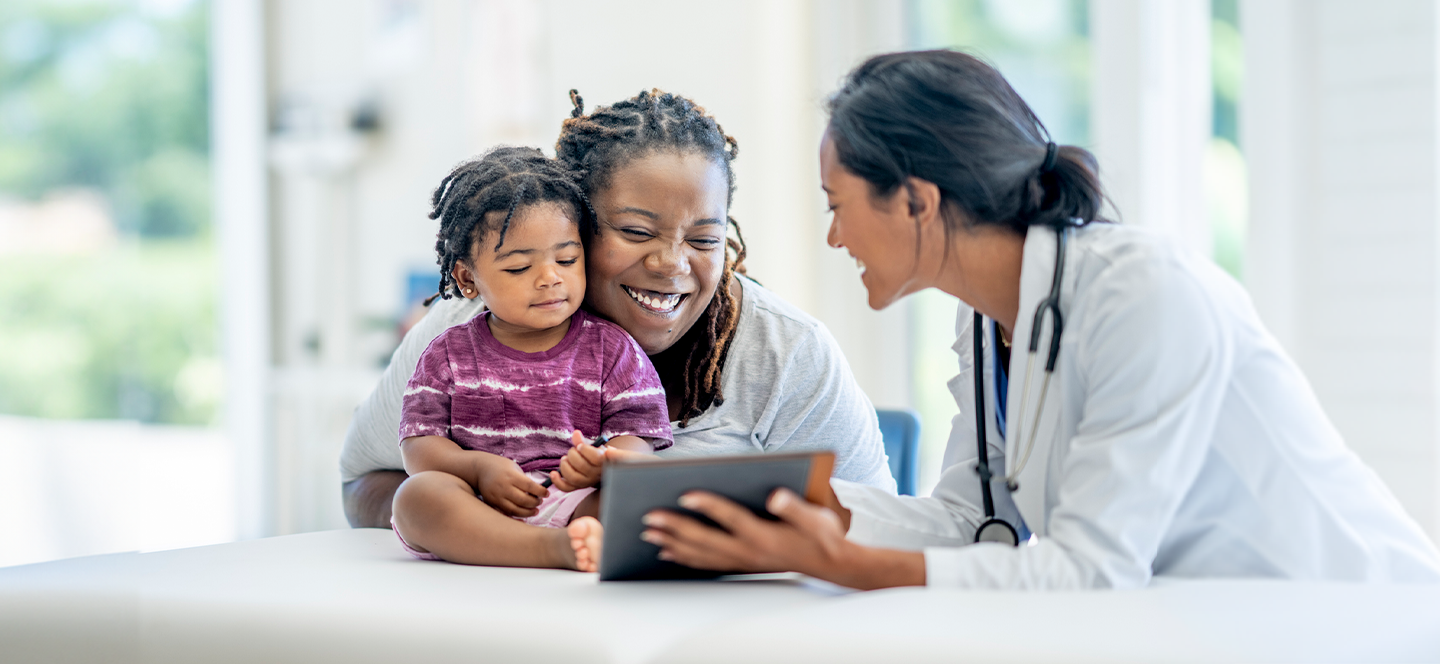
Providers
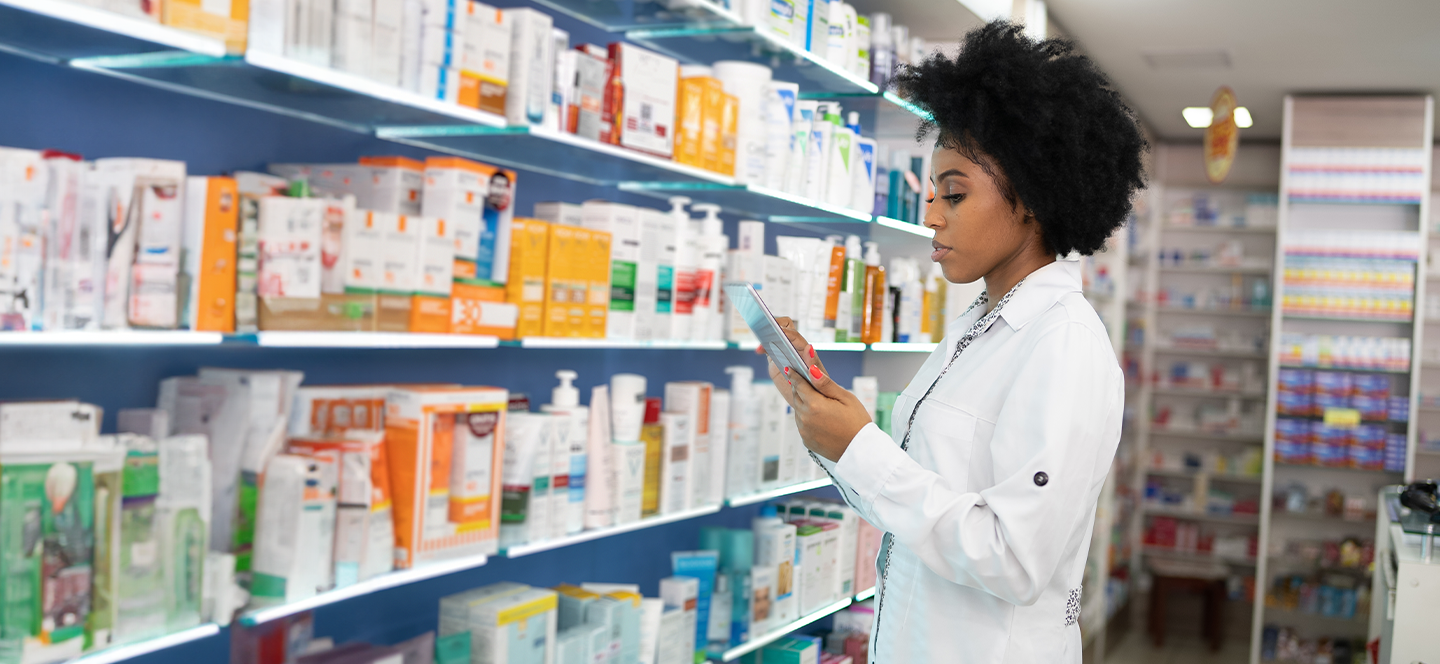
Pharmacy benefit managers
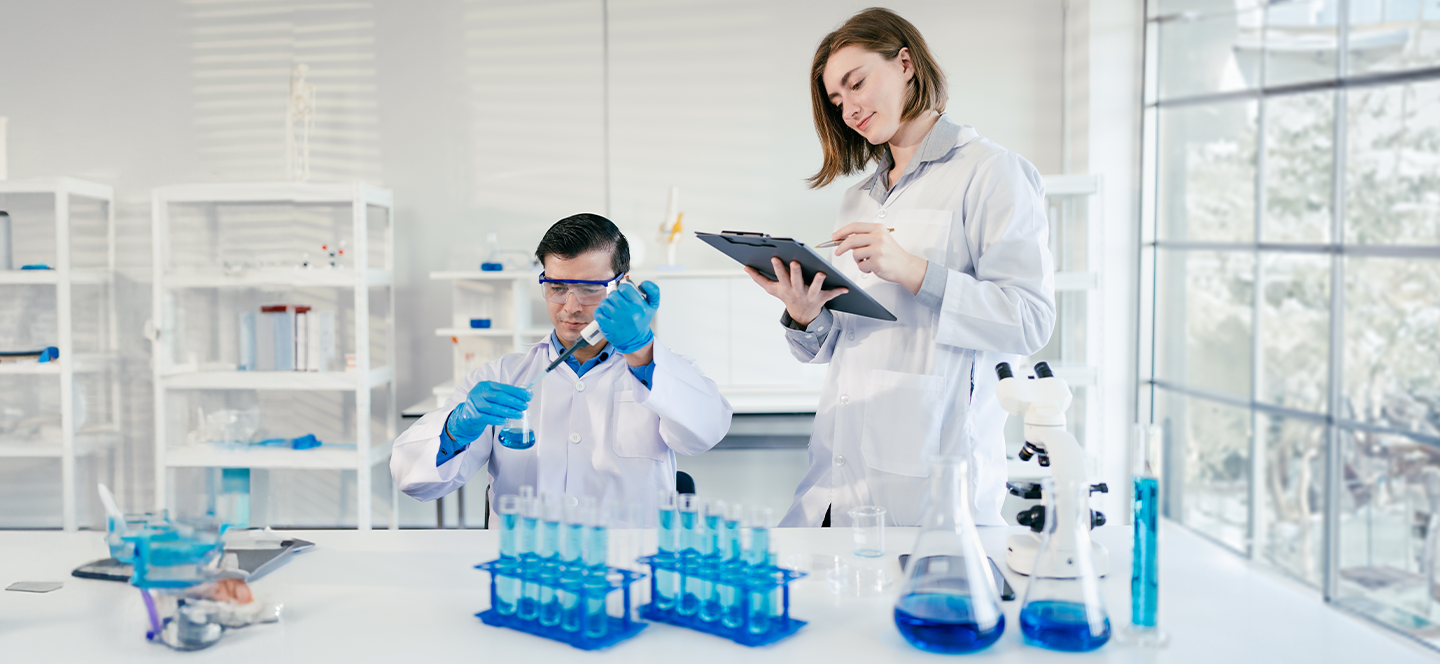
Life sciences
Health solutions
Evolving solutions for evolving needs
Why EXL
Applying our expertise in AI, analytics and cloud, we make sense of your health-related data, enabling you to improve outcomes, optimize revenue, and maximize profitability across the care continuum. Our digital dexterity and Human Ingenuity are the catalysts to solve complex healthcare problems.
We accelerate your ability to transform how healthcare is delivered, managed and paid. We amplify how quickly, efficiently and affordably you deliver quality care—enhancing your performance with nimble, scalable solutions.
Together, we will do this.
Get in touch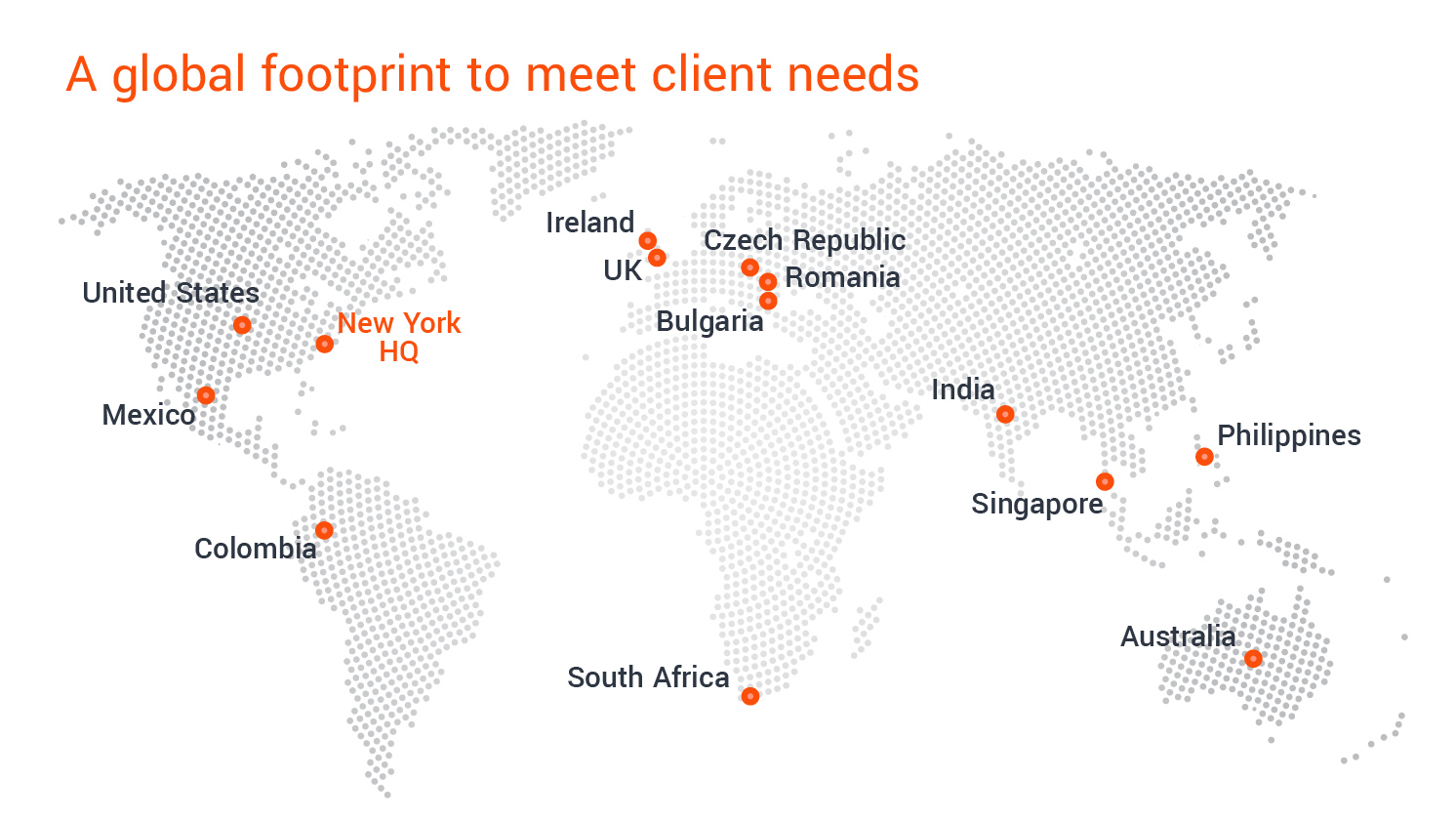
30+
hospital networks and IDNs
7
of the top 10 US payers
2
of the top 3 PBMs