A data-driven approach to counter insurance fraud
Fraud capture requires an understanding of non-intuitive patterns. Historically, the process has largely depended on the understanding and experience of claim handlers. Recent years have seen a significant development in identifying and tackling suspicious cases using internal and external data coupled with predictive analytics and proactive processes.
Kshitij Jain
Senior Vice President
EXL
We know that data and technology can help our expert call handlers enrich the decisions they make. So, we sought an AI solution that acted like a seasoned handler, to assist, rather than replace our experts, in capturing fraud.
Our fraud models, delivered in partnership with EXL, relied on a test-and-learn approach to capture the learnings from our most experienced handlers. By understanding the overall flow of a claims journey and working collaboratively across the claims, data, and change management teams in partnership with EXL, we were able to deliver significant savings, a better claims experience, business insights and team training material.
Robin Challand
Claims Director
Ageas UK
An agile fraud framework
Claims indemnity payout constitutes a major portion of costs for insurers. In recent years, non-life gross claims payment amounted to over £12 billion[1] in the UK, of which £8.3 billion was paid towards motor insurance claims. The overall average value of a motor claim was at £4,000, while the average motor insurance bodily injury claim cost £12,100[2]. Identifying fraudulent claims has been a key challenge for most insurers, with the situation deteriorating in recent years due to the evolving fraud landscape. According to the Association of British Insurers (ABI), the industry spends around £250 million each year combatting fraud[2].
An additional ABI survey of members revealed that the industry saw 96,000 fraudulent insurance claims worth £1.1 billion uncovered by insurers, with a rise in average value for fraud claims[2]. Motor insurance fraud remained the most common, with a total value of £602 million.[2] This suggests that fraudsters have evolved even during the adverse situation of the pandemic to increase average payouts for fraudulent claims. This highlights the need for a robust fraud detection ecosystem to increase fraud capture and protect innocent people by helping insurers lower indemnity spend.
While the industry developed in the ways it captures fraud, fraudsters have likewise improved their tactics. Insurers must create an evolving and sustainable ecosystem to capture current fraud trends while incorporating newer patterns.
Rethinking analytical interventions in fraud for incisiveness
An in-depth understanding and scientific evaluation of fraud detection incorporating the four central areas of data, people, process, and technology, is essential to design the best interventions for all stages of fraud identification and capture.
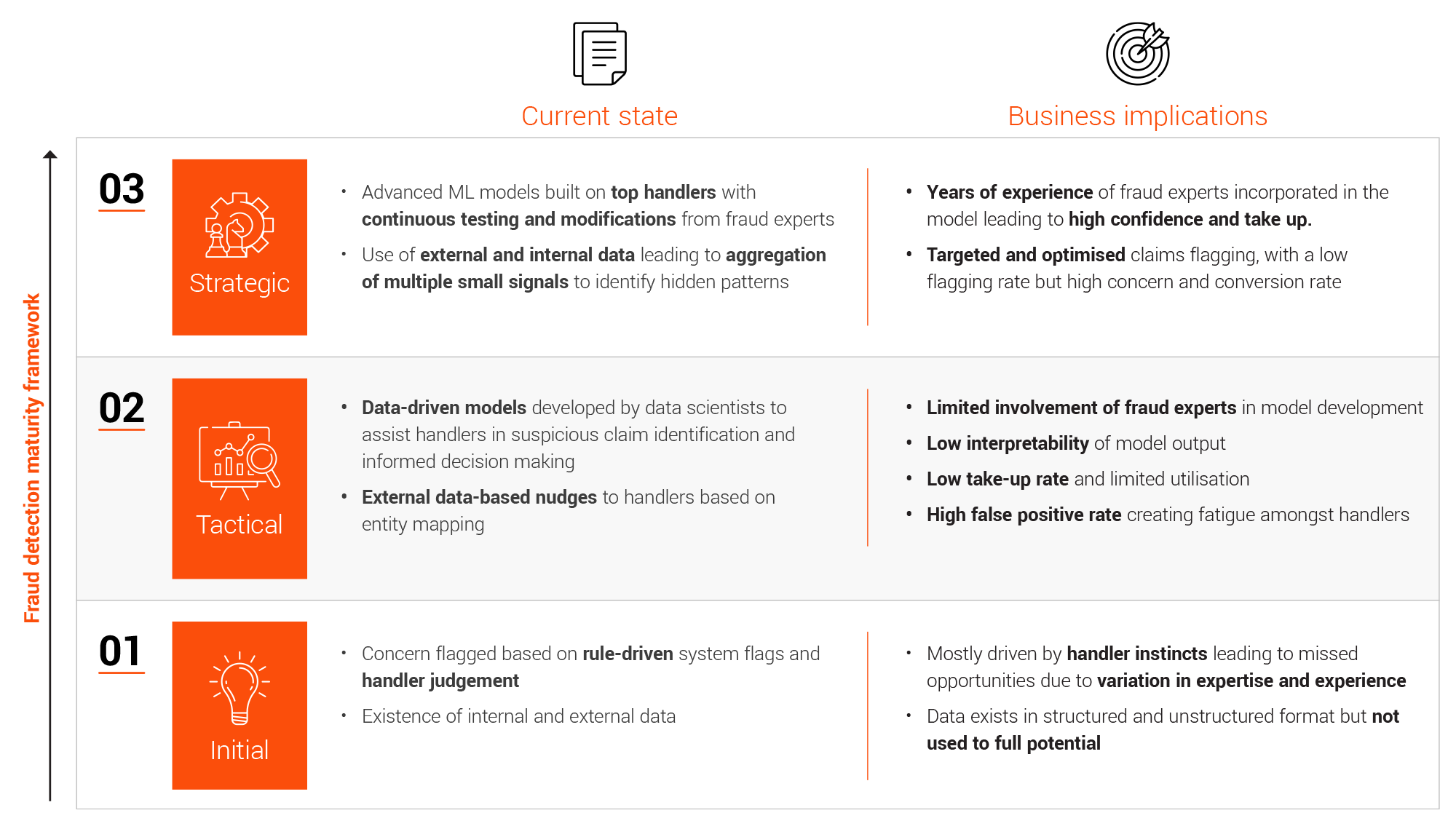
Insurers have evolved from fraud detection based on a handler’s judgment to identifying data-driven fraud patterns. EXL’s experience shows a move to this approach can improve fraud capture rates by around 5-10%. The changing approach of fraudsters and their as-yet unidentified fraudulent patterns now calls for a more strategic, sustainable approach.
EXL has developed and implemented its strategic fraud capture framework for numerous clients and provided significant tangible benefits:
a. Increased indemnity savings through improved fraud capture rate
b. Decreased operational expense through reduced false positives and more targeted fraud capture attempts
Insurers can meet these objectives by following a four-step strategy driven by effective use of data and analytics across the claims value chain.
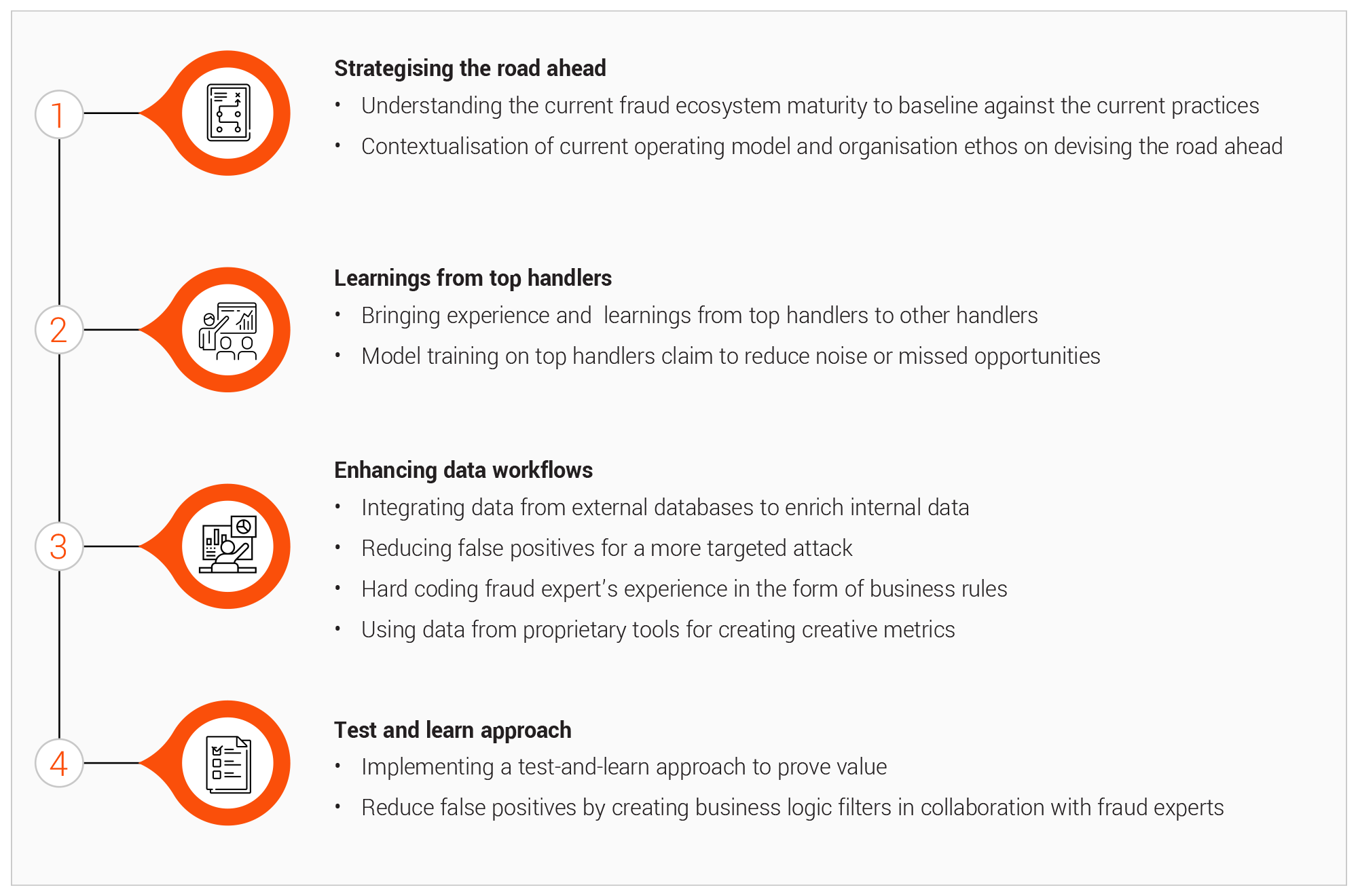
1. Strategising the road ahead Different organisation are at different levels of maturity, with different operating models and organisational approaches to identifying suspicious claims, investigating fraud, and capturing fraud. This makes it impossible to impelment a one-size-fits-all solution for every situation.
a. Understanding the current fraud ecosystem maturity:
Insurers must understand the current state to identify the major impact areas and strategise around it to optimise their efforts and maximise benefits. It becomes imperative to do year-on-year comparison and see past performance from multiple lenses. The concern rate and conversion rate acts as the two major indicators, along with indemnity savings, capacity utilisation and other soft indicators to understand how the past strategies have shaped the current fraud landscape and what can be a good course of action.
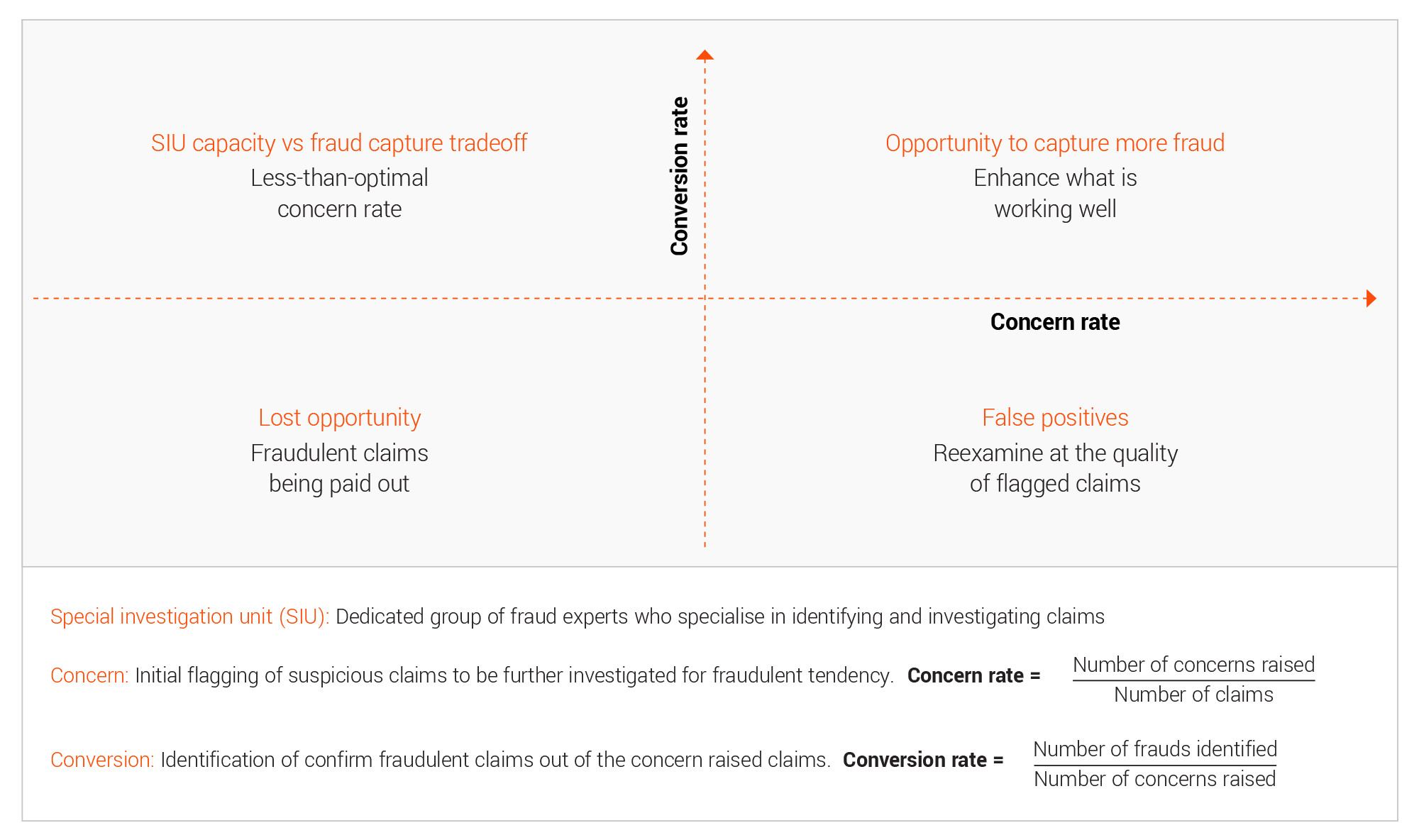
b. Contextualisation of current operating model and organisation ethos: The current fraud operating model and organisational ethos, like empowering handlers or creating an SIU focused on investigating fraud, can play a crucial role is designing the future roadmap. Each model has it own sets on pros and cons that must be taken into consideration.
a. Giving power to all the handlers: The overall claim journey has a lot of touchpoints. Fraud can be uncovered at any of these touchpoints, like the way a claimant interacts at FNOL, the documents a claimant shares during a claims assessment, or at other points. Since handlers are the frontrunners at all these touchpoints, placing power in the hands of the handlers can help upskill them in every aspect of claim handling and also create a sense of responsibility towards fraud identification.
b. Creating a separate SIU team: Creating a specialised team of fraud experts can help in detailed claim investigation since the team will look at all the scenarios and every minute details.
2. Transfering the learnings from the best handlers The experts involved in claim processing often have incredible insights into fraud capture. They bring years of fraud identification experience and are able to read hidden non-intuitive fraud patterns with ease.
Handlers normally plays a pivotal role in identifying concern. There remains a significant variation in handler performance due to experience, training, attention to details, and other traits.
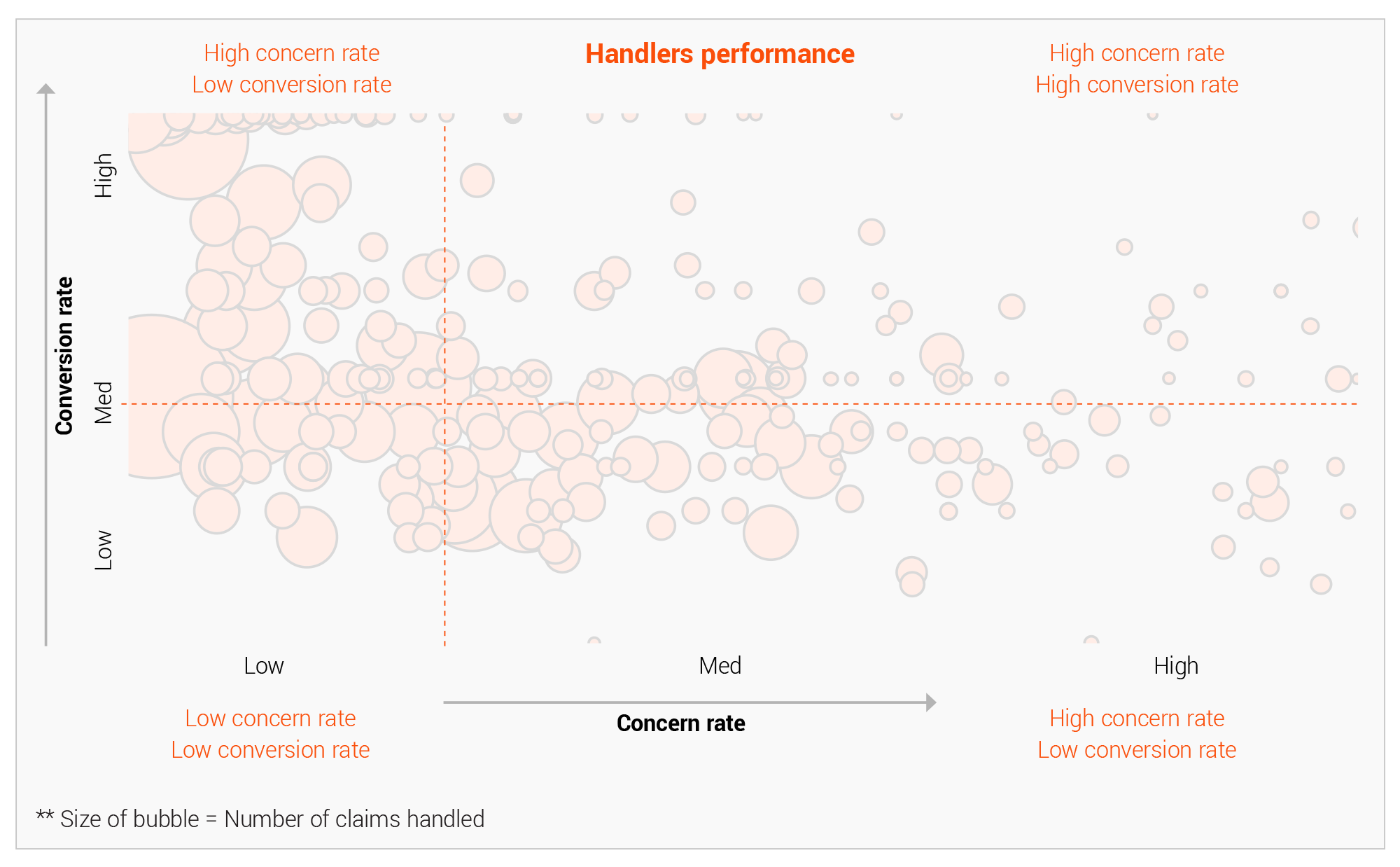
This knowledge can be learned and modelled in the form of system rules. This approach leads to a much better fraud knowledge and recognition system across the function than randomised self-discovery. Doing so ensures a proactive response to existing fraud patterns and the discovery of new ones across broader teams.
3. Enhancing data workflows and processes for decisiveness
Internal and external data sources provide insurers with opportunities to improve their fraud detection strategies. However, both types of data also present challenges. While internal data is quite diverse, it needs to be pulled together using a central data asset to create a single source of truth. External sources, on the other hand, presen unique cross-industry intelligence that is distinct and potent but must be extracted and integrated with the internal data elements.
a. Integrating various external data sources to internal processes Various forms of external data require different levels of processing to extract and utilise their intelligence:
1. Cross-industry data often requires entity matching and resolution for use with the internal data.
2. Open-source APIs like Ministry of transport (MOT) and Driver and Vehicle Licensing Agency (DVLA) require automated data sourcing and wrangling.
3. External text data are often unstructured and require text processing and natural language processing (NLP) solutions to extract information.
4. External documents and image data require optical character recognition (OCR) and intelligent character recognition (ICR) with metadata feature extraction.
Ideally, when external data sourcing and wrangling are sufficiently automated, it can encompass multiple areas of claims to aid with fraud detection.
b. Enriching the external data sources and creating value
Many insurers find the intelligence from external data is usually very noisy and has sub-optimal use in its initial state. Inadequate data quality checks exacerbate this issue. Enriching external data sources mainly entails:
1. Reducing false positives using system rule-based prioritisation and filters.
2. Entity matching, resolution, and data deduplication for proper linkage.
3. Choosing appropriate observation window to avoid bias from older data.
An insightful position-based view in terms of flag rate and conversion rate helps determine the current and future state of this enrichment exercise.
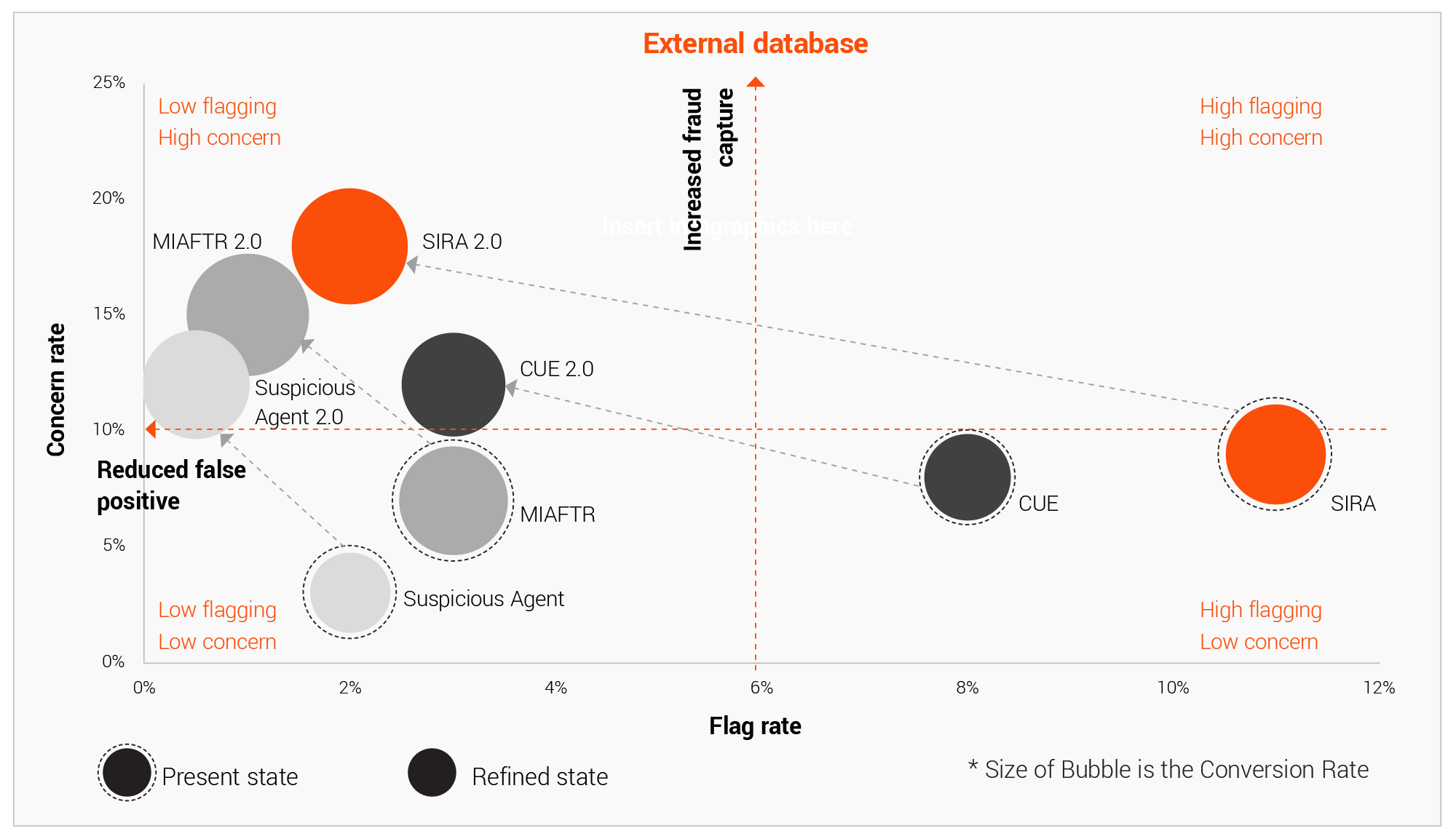
After augmenting the data, fraud models should include two main types of intelligence:
1. Entity-based intelligence: Identifying the fraudulent claimants previously captured in various external databases through information such as their name, surname, addressd, telephone number, and vehicle registration number. This intelligence plays a crucial role in identifying hard fraud, or premeditated and deliberate fraud attempts often involving professional fraudsters or fraud rings.
2. Context-based intelligence: Identifying the fraudulent patterns like delays in advising a claim, claims advised close to policy inception, and other identifiers. This intelligence plays a crucial role in identifying soft fraud, which is opportunistic and often done by exaggerating damages.
The above clues will enable better and swift fraud capture when proactively prompted to handlers, thus maximising concern and fraud conversion rate.
c. Creating a single source of truth through data lakes
Data residing in various sources and within different functional areas helps business users by providing valuable insights. This can include information about customer demographics and vehicle attributes found in policy documents, customer behaviour patterns from external databases like SIRA and Claims and Underwriting Exchange (CUE), and current claim circumstances from claims data. It is quite crucial to bring all this data together in a connected ecosystem to create a single source of truth that is updated in a timely manner to unlock the real value of identifying fraud patterns.
Building a data lake helps insurers bring together data present in multiple formats like structured data, streamed event data, unstructured data from multiple sources and present within various functional areas. Doing so creates a robust data management process while adhering to requirements around data privacy and the protection of personally identifying information while simultaneously ensuring security, auditing, and privacy requirements.
4. A test-and-learn approach towards fraud management
The scientific approach to any fraud model involves inferring from historical trends, the newfound knowledge from external and internal sources, and using advanced analytical techniques. Incorporating an exhaustive and empirical testing framework is essential to realise benefits from the modelling and implementation journey.
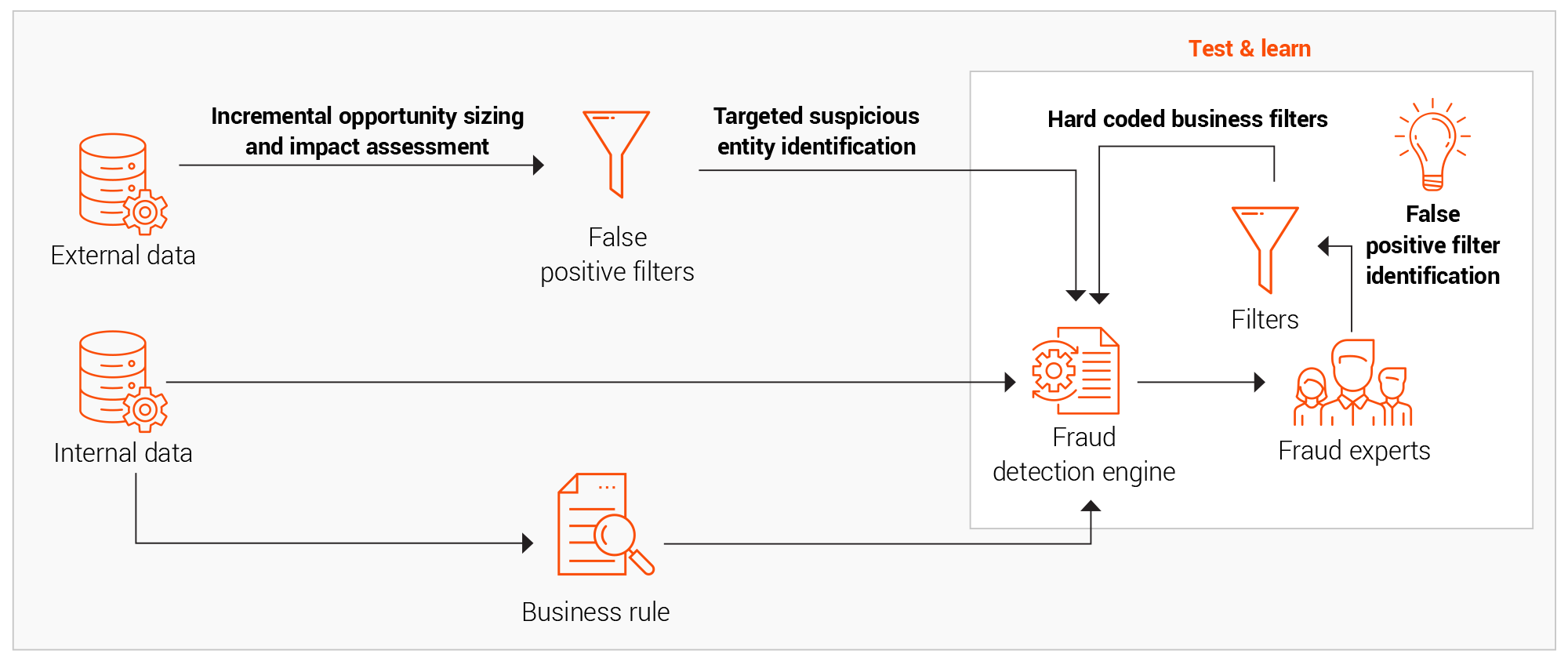
Proof of a value-based test-and-learn framework
The importance of taking a test-and-learn approach to fraud analytics arises from the changing nature of fraud. Its advantages include:
1. High solution adoption due to an evolving test and learn framework benefitting from increased stakeholder involvement in model development, model testing, and model optimisation through false positive reduction.
2. An early view of the benefits resulting in high confidence in the approach, leading to greater end user satisfaction.
3. Mapping business logic and fraud expertise into the model development process collaboratively between fraud experts and data scientists.
4. Identify drivers for genuine claims reduces false positives.
Improvements from a test-and-learn approach to fraud modelling New models and solutions often face many implementation and working challenges, which are resolved by testing and learning from them. The new proposed framework provides far-reaching benefits:
1. Production improvements
a. Smooth production processes and experimental refinements contribute to sustainability and focus on high marginal benefit areas
b. Low turnaround time for changes at any point in the model flow
2. Model efficacy and longevity
a. Factoring in concept drifts from events across timelines due to continuous improvement is a core concept in the test-and-learn approach.
b. Modular models are easily replicable both as a group and standalone. Filters provide more insightful control than black box hyperparameter optimisation.
c. A stratified view of the output leads to better deep-dive investigations and priority-based recommendations for optimal resource use.
3. Reduction in technical debt
a. The framework reduces technical debt due to extensive knowledge collection and collaboration.
The continual quest to derive value for insurers fighting fraud requires a blend of proactiveness and pragmatism. This agile fraud framework empowers process transformation through data and analytics interventions coupled with a test-and-learn approach, shifting the value proposition for insurers from proof of concept to proof of value.
This test-and-learn framework based is a crucial step forward in fraud model utility and improvement. Shifting focus from just theoretical model accuracy to continuous model and process improvement, stakeholder engagement, and technical excellence combined with agile principles can ensure long term success. This can help increase fraud capture rates by around 5-10% while simultaneously keeping false positive rates under control. EXL has helped clients realise significant tangible benefits through improved fraud capture and better SIU utilisation, with in excess of four-to-six times the initial investment.