Navigating the path to data driven finance
If there is one thing the COVID 19 crisis has underscored both across and within industries, it is that the top performing companies in the post-pandemic world will be data-driven.
This is not surprising. Rising competition, the battle for customer loyalty and attention, shorter business cycles, and a rapid shift to digital for everything from product and service innovation, distribution channels, and business operations puts unprecedented pressure on businesses. If driving profitable growth was hard before, it is even harder now as organizations find themselves having to pivot rapidly and frequently in response to a constantly evolving and disruptive market environment.
To successfully compete and profitably grow in this challenging environment, top performing datadriven businesses use data and analytics in ways that consistently produce better decisions, automate key processes and enable personalized digital interaction will drive higher economic value, customer loyalty, and enterprise efficiency, thereby creating sustainable long-term competitive advantages. Perhaps no firms exemplify this better than Amazon and Netflix, but while they are best known examples, they are certainly not the only ones. Virtually every industry has seen the rise of nimble, agile, data-driven start-ups challenging incumbents.
To successfully make this pivot, organizations are turning to their CFOs for help. In their role as strategic partners, CFOs are expected to support their businesses in uncovering new sources of value through deep insights while simultaneously taking rapid action by driving objective, granular, and fast decision support.
To successfully deliver to these expectations, CFOs need to transform finance to become truly data-driven.
As custodians of their organization’s data, CFOs are in the best possible position to accelerate the shift to data-driven change, not just across finance, but across the entire organization. From day-to-day transactional decisions, such as how to improve working capital, to core strategic decisions, such as how to allocate capital or evaluate targets to maximize enterprise value in M&A valuation, data has the potential to become a valuable enabler.
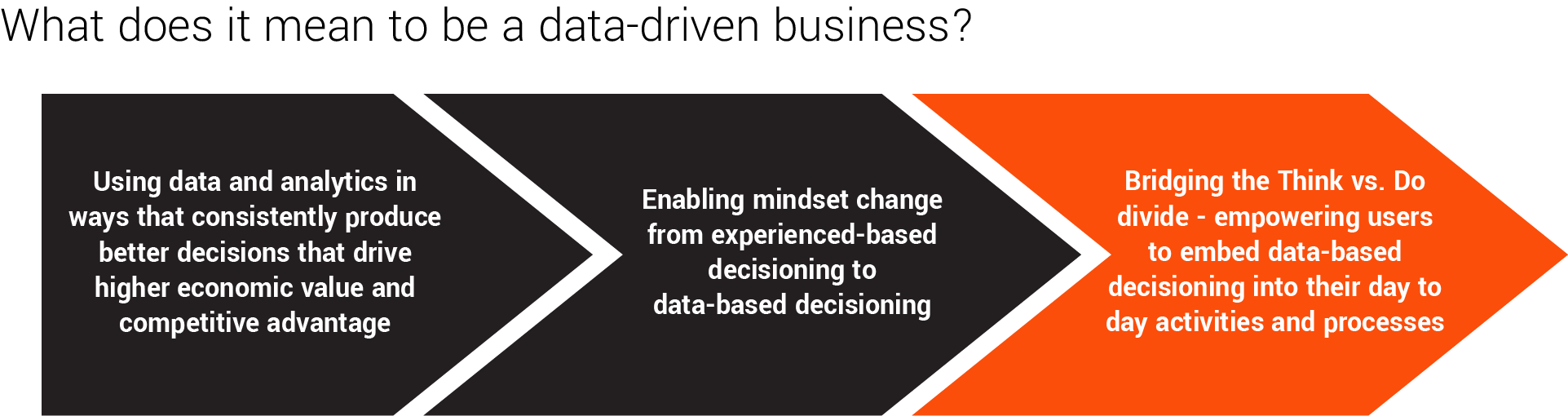
The path to becoming data driven is hard
A Big Data and AI Executive Survey conducted by NewVantage Partners in 2019, showcases a decline in companies who identify themselves as being datadriven from 37.1% in 2017 to 31.0% in 2019, despite the accelerating pace of investments in big data and AI1
Firstly, it is hard to get organizations to quantify the value of data driven decisioning. The proof of value and the true measure of success is hard to quantify, since the quality of the outcome is determined not only by the quality of decisioning but also by the quality of the subsequent execution. This is a hurdle that often prevents many executives from buying into the concept, even when presented with success stories from other parts of the organization.
Secondly, data and analytics initiatives are often hampered by a lack of investment in modern technology infrastructure, the absence of current analytical tools, and data-related challenges.
Finally, data and analytics are advanced competencies that have only recently entered the mainstream. Figuring out the right balance between hiring from outside and building from the inside is a lot harder with no existing blueprint.
Collectively, these challenges highlight why a majority of organizations struggle to become data driven.
To successfully address these challenges companies need to go beyond the technology transformation and execute on the following three core transformations – culture, data and talent.
37.1% in 2017 to 31.0% in 2019
a decline in companies who identify themselves as datadriven.
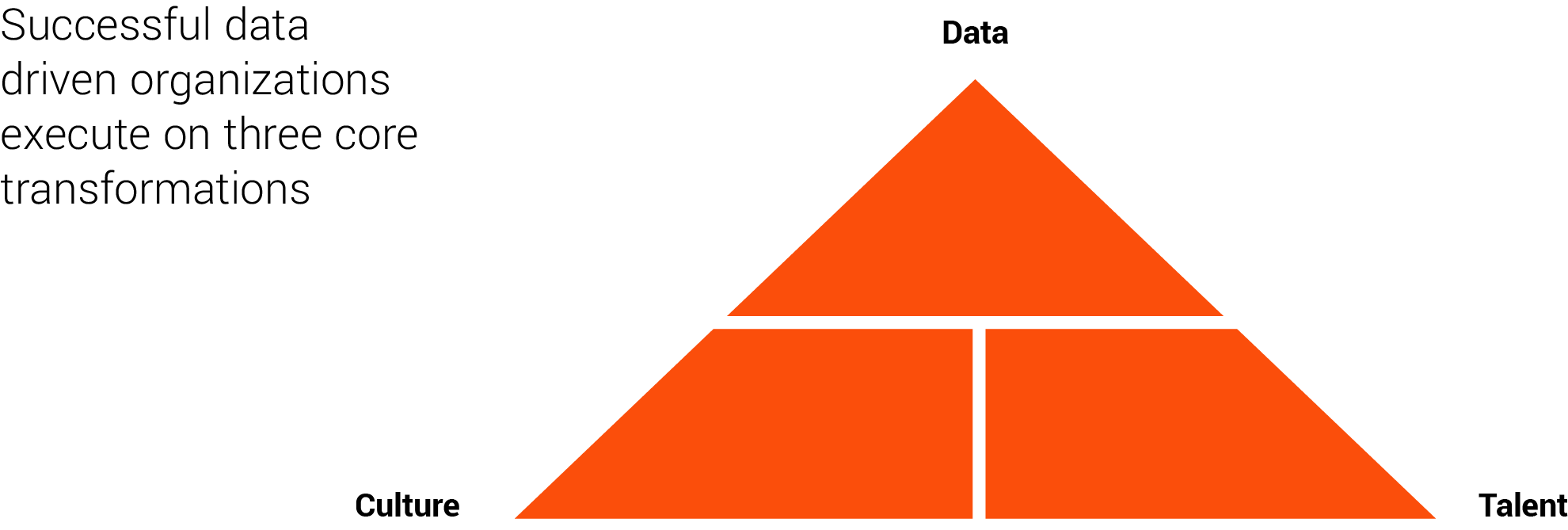
"We’re focused on shifting our perspective and changing existing mindsets in building a data-driven decisioning culture. We’re moving beyond the traditional stewardship role within finance and becoming better business partners. We’re utilizing all the data that we have access to in the finance department, to become better at anticipating business changes and opportunities. Data is allowing us to be become more flexible and nimbler."
Mickey Coontz
Vice President, Head of Finance Data Strategy,
New York Life Insurance Company
Creating a Data-Driven Decisioning Culture
In the CFO organization, as in other parts of any business, a data-driven culture is the result of a fundamental and foundational change in the collective human behavior of an organization.
There is a tendency to implement technology such as data lakes and reporting tools in hopes that they will empower data-driven finance. While technology may work as an enabler, it’s seldom the whole answer. Ultimately, it is humans who run the show. The way they think, adopt the change, and innovate will play a big role in the success of adopting data-driven decisioning.
With any major change or cultural transformation, the tone at the top matters. Therefore, frequent and consistent top-down communication on the importance of a datadriven approach and support for ongoing initiatives is very important, as is the need to make the necessary investments in technology, people and training.
But perhaps the most important first step is to recognize that getting the whole organization to learn and adopt an entirely new way of thinking, problem solving, and decisioning is like building new muscle. To be successful requires consistent effort over long periods of time, persistence in the face of inevitable setbacks, and, most importantly, clarity in terms of why it is worth the effort.
Answering the “why” or “what’s in it for me?”
Unless organizations can clearly explain to their employees why they need to embrace data-driven decisioning and make the effort to learn new skills, it will be extremely difficult to sustain any initial momentum that the first few highprofile pilot implementations might create. While success stories from other companies, and even from other functions, within the organization are helpful, nothing works better than customized proof of value use cases for each area of the organization. While it does create a somewhat slower rollout since each functional area starts with one or two use cases, it is more sustainable as it creates much higher engagement and support from within.
Build critical mass and momentum
To build on the success of the initial proof of value use cases, it is important to create a pipeline of initiatives running across and within functions. Having a pipeline helps sustain and build on the initial momentum while helping teams get better with each project, applying learnings and success factors in subsequent use cases.
By carefully curating the mix of use cases, organizations can ensure they are touching not just senior decision makers or highly specialized functions like FP&A and corporate finance, but also front line employees in transactional functions like accounts payables, billing, sales support, customer service, and receivables management. The advantages of such an approach are easy to see and can go a long way in bridging the “think vs do” divide between senior management and the frontline. The engagement and support of the frontline employees in turn creates a flywheel effect, generating more use cases and building sufficient critical mass to become a selfsustaining culture.
CFOs can play a key role in building this culture in two ways. First, they can champion this directly within the finance function, which is usually an ideal place to start given that most finance professionals are comfortable engaged in some form of data-driven analysis and decisioning already.
Second, finance can and should take the lead role in helping drive the proof of value pilots, particularly around business case development and building the initiative pipeline.
A pragmatic approach to the data challenge
As organizations extend and expand their data and analytics initiatives across the organization, they inevitably run into challenges around data quality and availability.
It is one thing to be able to create high quality data assets in the highly controlled and secure environments of small scale pilots. It’s a completely different thing to get the same quality, accessibility and control over data when it is not only much larger in scope, but also spans different functions, processes, systems, and geographies. This raises important and difficult questions.
Do employees know what datasets they need and how they can access them, especially if they’re in different siloes? Do they trust, or even know the lineage of where it came from, and what changes may have been made to it? Is the data good enough for its intended use? Can it be integrated with other data sets? What are the regulatory or privacy related implications if data sets are used for different purposes?
Understanding and managing the challenges of data quality, security, privacy, availability, and accessibility as one makes the transition from pilot phase to making data and analytics part of business as usual is therefore critical. Without proper governance and a clear approach, standing up a data-driven organization can quickly become an expensive non-starter.
In EXL’s work with scores of clients over the last decade or so, two things have worked well in addressing this challenge.
Start small and focus on the most important data first
When organizations encounter less than perfect data, their first instinct is to stop initiatives in flight in an effort to fix it all. Though well intentioned, such diversions are neither practical nor realistic. As Steve Dine, Global Head of Data Management,
EXL, notes, "In any data-driven decisioning initiative, companies should be focusing on fit for use, not perfection".
It is important to start small, focusing on the most important use cases first. For these initial use cases, organizations should clearly identify the specific data that they need at a granular level. Doing so helps to ring fence data requirements and makes security, quality, and accessibility management much more bounded. The other benefit is that it allows organizations to limit any data quality fixes that are absolutely necessary to only those datasets (and within those datasets, to specific attributes) that are important.
As an example of what this looks like in action, the procurement team at an industrial manufacturer needed to model the impact of payment terms extension on their liquidity under various scenarios during the early days of COVID-19. However, their customer master data was not pristine. Fixing the customer master data, including deploying a Master Data Management solution would have taken over a year. Instead, the client focused on the top 100 customers that accounted for 80% of their revenues. They found that not only was the data quality for the top 100 customers much cleaner than the average, but that they really did not need too many critical fields from the customer master. As a result, the clean-up effort took only a few days, and the client was able to deploy the analytics program without delay.
"In any data-driven decisioning initiative, companies should be starting small, and focusing on governance, not perfection. We need to separate the signal from the noise because not all data has the same level of value. And we don’t necessarily want to store it all. Nor do we need to cleanse it before determining whether it’s useful. We can get the data in much faster for users to consume if we’re not so worried about ancillary attributes that maybe aren’t as important as they once seemed."
Stephen Dine
Global Head of Data Management
EXL
Establish a Data Strategy and formal Data Governance discipline right from the start
As data and analytics initiatives gather momentum across the organization, establishing the right data strategy and data governance upfront is critical.
Without a clear data strategy, managing the accessibility, availability, security, privacy, and ongoing data quality requirements can become complex very quickly in this environment. Establishing a data strategy roadmap will require organizations to assess their immediate and longterm data and analytics use cases across functions and processes, and understand the gaps within the current systems, technology, and information landscape. This will allow organizations to see potential roadblocks upfront and develop the right strategies and tactical plans deal with them.
Similarly, it is important to establish common standards for data management, security and privacy controls, and data ownership and lineage, especially as data spans across organizational siloes. This applies not only to data within the organization, but also to external data that might be brought in from time to time. CFO’s must partner with their data management teams and chief data officers (or CIOs) to ensure there is clear alignment of objectives, policies, and practices in this regard.
Without sufficient and rigorous data governance, the democratization of data is difficult to implement. As data is accessed and used across the organization in a variety of forms and for myriad purposes, clear rules must govern how it can be accessed and what limits are to be placed on its usage.
"Technical skill sets are important, but we emphasize the soft skills more. When we look for talent, we look for people who are willing to learn. People with a growth mindset, who are able and willing to accept change. We’ve found that it’s easier to teach someone who’s willing to be taught than it is to take someone who may have the advanced technical skills, but who doesn’t really know how to utilize them when it comes to collaborating."
John Sheehan, Sr. Manager
Vendor Risk Management and Global User Access Management
Johnson & Johnson
Addressing the Skills Gap
In order to realize the goal of becoming a data-driven organization, CFOs must look at their talent strategy in a holistic manner. Addressing the skills gap usually starts with building the technical capabilities around data management, analytics, and tools and technologies needed to implement different use cases such as data visualization platforms, data profiling, and scenario modeling solutions.
Most companies start by expanding their current data and analytics teams (as these are often two different teams), and hiring a combination of data scientists, analysts, and architects. While this is a great starting point, it isn’t sufficient.
Being truly data driven implies driving data-based decisioning to frontline employees. This is by far the largest group of data consumers on nearly all organizations. This means that having all data and analytics controlled centrally from a center of excellence is not the answer. CFOs must find practical ways to engage and train the rest of the organization to adopt this approach.
Identifying web-based training programs on data and analytics, and making them available on demand to employees, is a good way to get employees to sharpen their technical skills. However, often the bottleneck in the adoption of a data-driven mindset is that many frontline employees simply do not have sufficient experience or understanding of data-based decisioning and how it applies specifically to their roles.
To solve this, organizations need to tailor and customize their training programs to the specific needs of their employees. Start with the goal of increasing the data literacy of the organization by defining the data and analytics training needs in the context of their roles.
This means identifying what is important and why, what specific skills they will need, and the optimal technical background they need in order to be effective. The last point is especially pertinent - go too shallow and people will come away without a clear grounding in the first principles, go too deep and the learning curve can become too steep.
From a training perspective, surprisingly, this can initially mean a lower emphasis on technical skills and a much greater emphasis on developing an analytical mindset and understanding how to use data objectively for analysis and decision support. Done correctly, and supplemented by the relevant technical training, this approach can create much greater impact as employees begin to adopt new ways of problem solving driven by data, which in turn creates a virtuous cycle promoting a deeper understanding of the business and underlying value drivers
The Power of reciprocal mentoring
Reciprocal mentoring has proven to be a good way for organizations to create this virtuous cycle. This involves pairing data scientists and data specialists with operations, sales, marketing, HR, and finance employees to collaborate on the design and implementation of different use cases. This collaboration helps the business professionals learn new analytical techniques and gradually evolve a data and hypothesis driven problem solving mindset. For their part, the data scientists get a strong grounding in real world business challenges and constraints, thereby helping temper their technical skillset with valuable business context. As the CFO of a large pharmaceutical company that implemented reciprocal mentoring in the finance function put it,
"I don’t expect that my team of accountants will become data scientists overnight, but this will help them to work with and see the opportunities made possible by a data and analytics led approach".
Not everyone will find thinking in this different way easy. In this sense, a holistic employee learning and development program is an important aspect of addressing the skill gap, in addition to hiring specialists from outside the organization. It’s a journey with a hidden advantage: in working to train the CFO organization, data scientists get to understand the business better. Ultimately, companies benefit by creating a new breed of hybrid analytics and finance professionals from both departments.
Working with a Trusted Partner
Working with a trusted partner like EXL can bring skills and experience to the data-driven finance journey, helping to accelerate the development of a data culture, while also bringing pragmatic, hands-on experience to establishing data frameworks and filling skill gaps. A trusted partner can also be the solution to initiative fatigue, helping to move beyond any initial progress to provide critical mass and momentum, as well as helping to drive desired business objectives and improved outcomes.
It’s a complex journey, and one that requires positive reinforcement every step of the way. However, with the right partner, the CFO organization is well positioned to begin turning information into insight and to capitalize on new data-driven opportunities on the horizon.
Acknowledgement
The authors would like to thank Mickey Coontz, John Sheehan, Stephen Dine and Nikhil Mathur for their contribution to this white paper.
References
Written by:
Narasimha Kini
Senior Vice President and
Global Business Leader,
EXL Emerging Business Unit
Nikhil Anand
Vice President and
Client Executive,
EXL F&A Center of Excellence