The future for utilities starts with EXL today
Utilities companies worldwide are seeking to preserve profits in a heavily regulated and inflationary marketplace, largely using aging infrastructures, while catering to the increasing demand for “net zero” emissions from customers and regulators. Customers at every level are struggling to pay their bills – even as they demand more fluid customer experience and energy conservation at all costs. Has there ever been a better time for transformation in the utilities sector? EXL offers the domain expertise, digital savvy, and robust analytics capabilities you need to take charge now.
Who we serve
EXL's advanced analytics, generative AI and cloud capabilities help organizations drive actionable insights at speed.
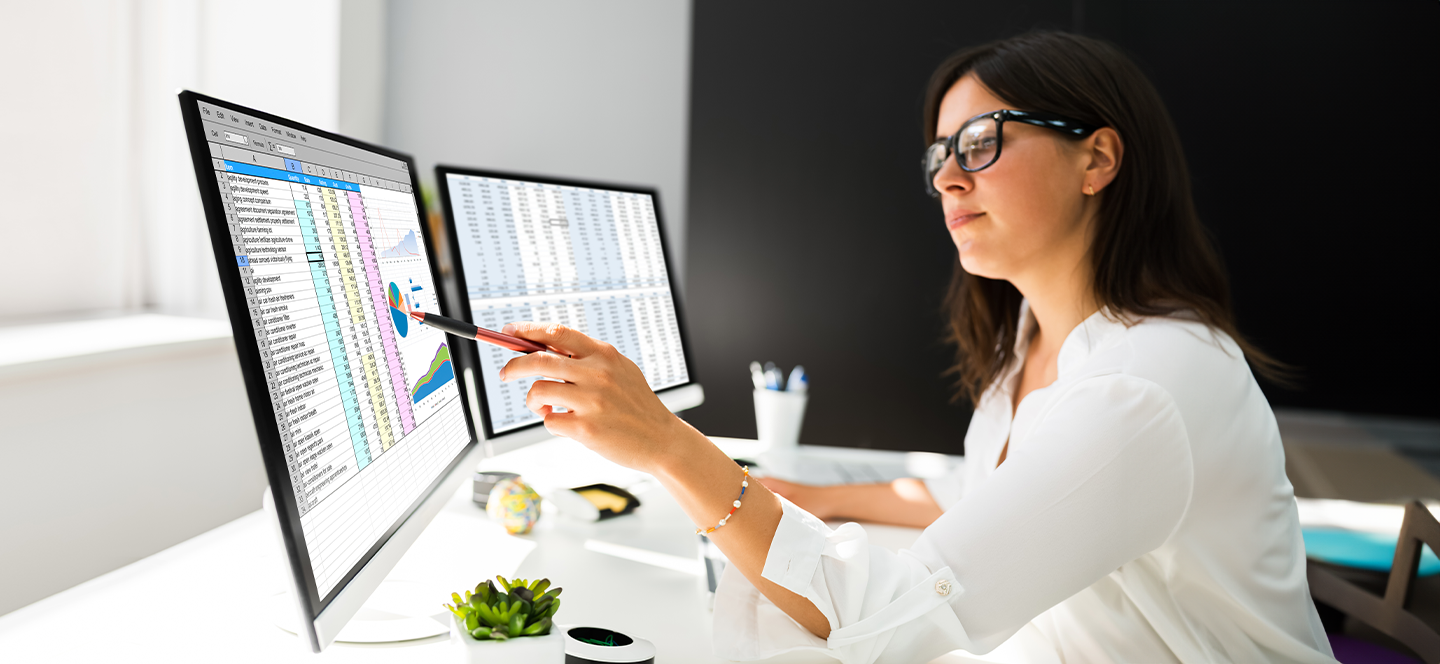
Field and metering support
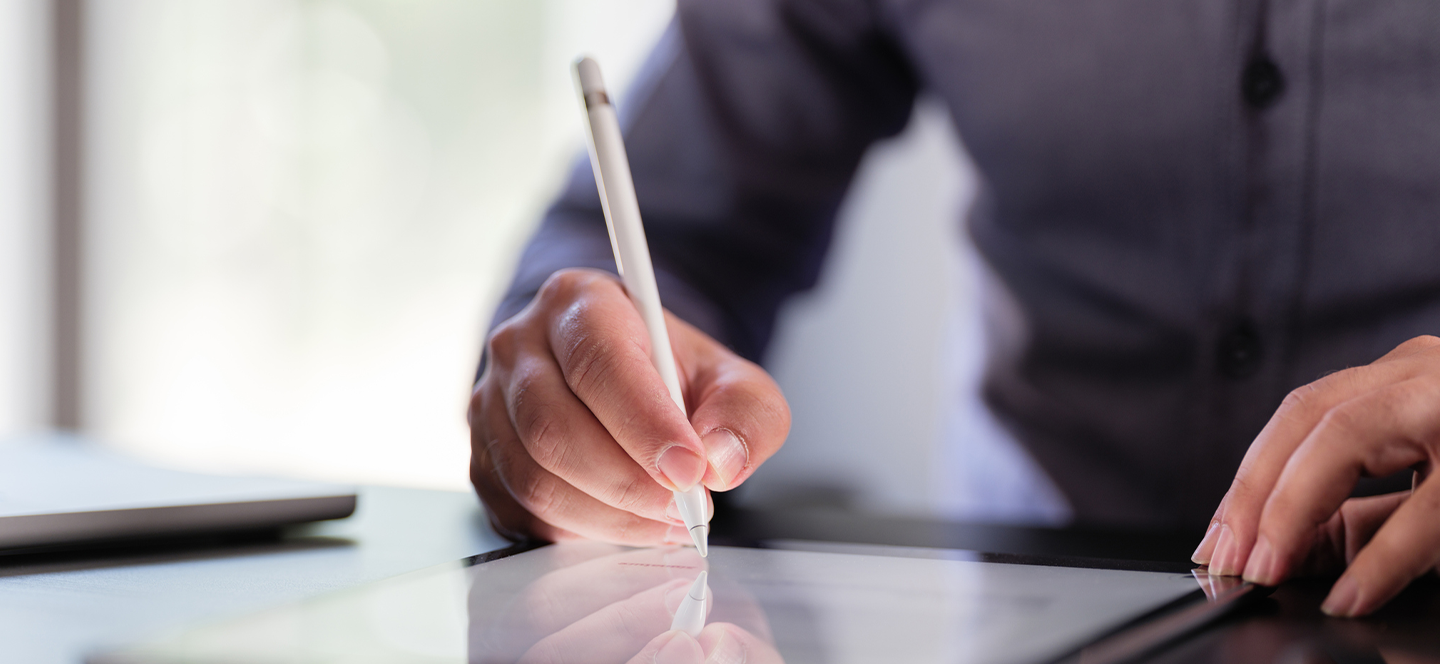
Net Zero
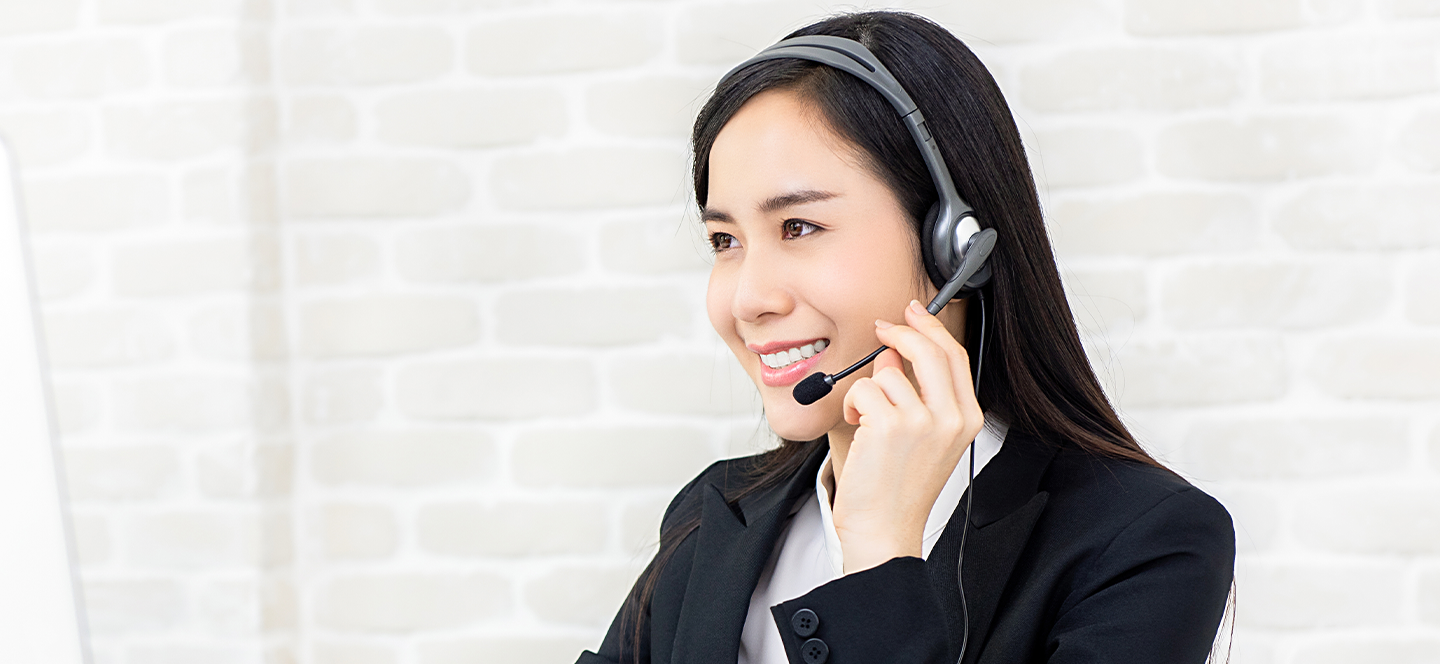
Retail operations and customer experience
Utilities solutions
Evolving solutions for evolving needs
Why EXL Utilities
EXL has served the utilities industry for more than 20 years, devising practical solutions that make sense of data to make human efforts more valuable, meaningful, and rewarding.
Our global team, numbering more than 6000 resources, stands ready to assist in building more customer-centric and compliant operations, deeper insights through analytics, and lower cost through our AI-powered digital solutions that have returned more than $90M in value to our clients worldwide over the last 5 years.
As effective partners, we have received accolades across the industry and continue to build our client and knowledge base with every engagement. Be proactive and succeed. Take a closer look at EXL.
Get in touch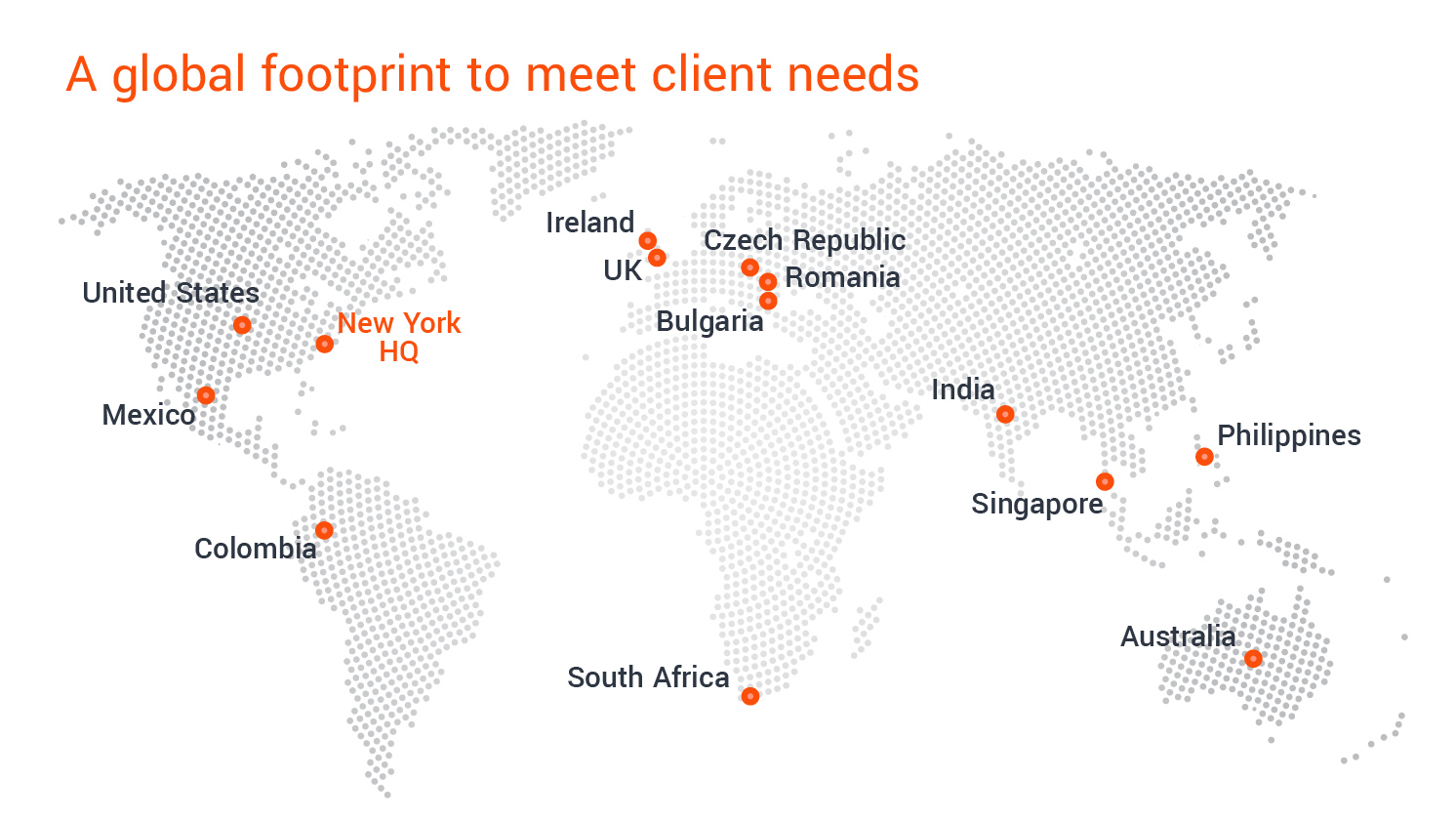
Leader
in Everest Group Analytics and Artificial Intelligence (AI) Services Specialists PEAK Matrix® 2024
Business Services Programme of the Year
in Global Sourcing Association (GSA) UK Awards 2024
Major Player
in IDC MarketScape for Artificial Intelligence Services 2023