Helping insurance companies win with data and AI
Superior customer experiences. Profitable growth. Greater speed-to-market and efficiency. EXL uses data, analytics, domain software, and artificial intelligence to make these goals achievable for global insurers, reinsurers, brokers, and insurtechs.
By combining our cloud-first digital insurance software solutions and industry expertise with generative AI, machine learning, advanced analytics, and platforms, we enable insurance businesses to transform operations and embed artificial intelligence into workflows.
Together, we partner with our clients to implement a flexible, customer-focused approach that delivers end-to-end outcomes.
EXL impact
Powering the success of global industry leaders
Our AI-powered cloud solutions and industry expertise help insurers unlock the full potential of their data, transforming operations and improving business outcomes.
430+
insurance clients
9
of the 10 leading US insurers
3
of the 5 leading UK insurers
3
of the top 5 brokers
Who we serve
We partner with clients to deliver data-driven insurance tech solutions
Insurance solutions
Evolving solutions for evolving needs
"EXL has been an exceptional partner for our Program Management Office (PMO). Their Project Management and Business Analyst support have been instrumental in the successful completion of cross-functional and strategic initiatives throughout our organization. Their collaborative and flexible approach, combined with their industry knowledge and experience, has enabled our PMO to become a trusted and valued department within the company. We greatly appreciate their attention to detail, professionalism, and commitment to excellence, and would highly recommend their services."
Adam Roth | Fortitude Re, Assistant Vice President PMO and Portfolio Management
Why EXL insurance
With a deep understanding of the industry developed by serving over 430 global insurance clients, EXL knows how to make sure your company stays future-ready and can adapt to changing trends and customer expectations.
Our analytics- and AI-led insurance technology solutions lets you pinpoint opportunities for growth, gain a holistic understanding of the market, and back all of your decisions with data.
EXL acts as your trusted partner to drive the results you’re looking for, whatever they may be.
Get in touch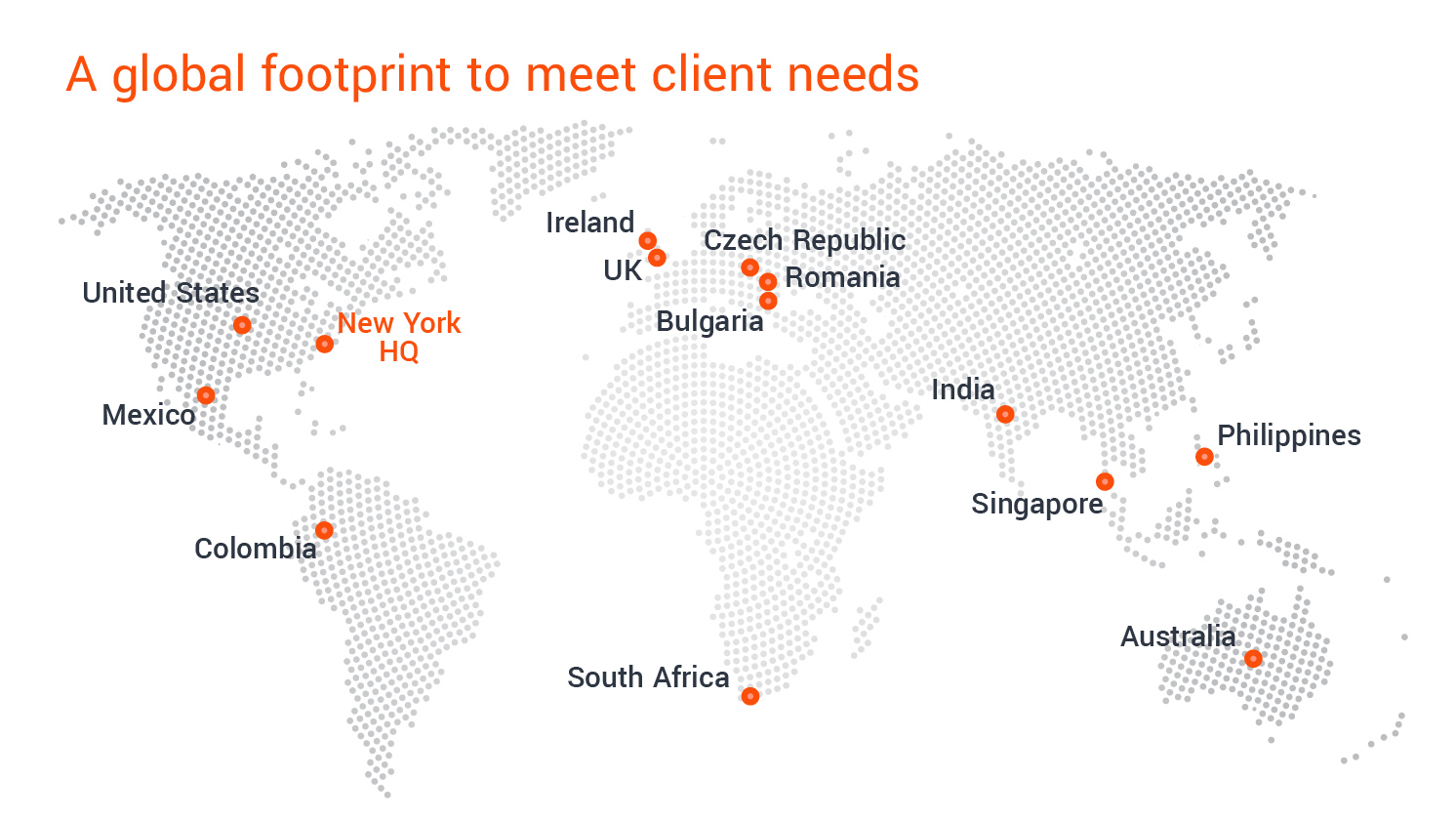
Licensed as a TPA
Capable of providing services in all 50 states & DC