Navigating your journey with seamless solutions
The transportation and logistics industry faces several challenges, such as global supply chain disruptions, fluctuating fuel costs, increasing customer expectations, and cargo theft, to name a few. Any of these can impact efficiency, sustainability, and profitability. These challenges are influenced by various factors, including technological advancements, regulatory changes, economic conditions, and environmental concerns. Fortunately, EXL has overcome these challenges for clients in the past and stands ready to empower your business for brilliant execution across your extended supply chain.
Who we serve
EXL's advanced analytics, generative AI and cloud capabilities help organizations drive actionable insights at speed.
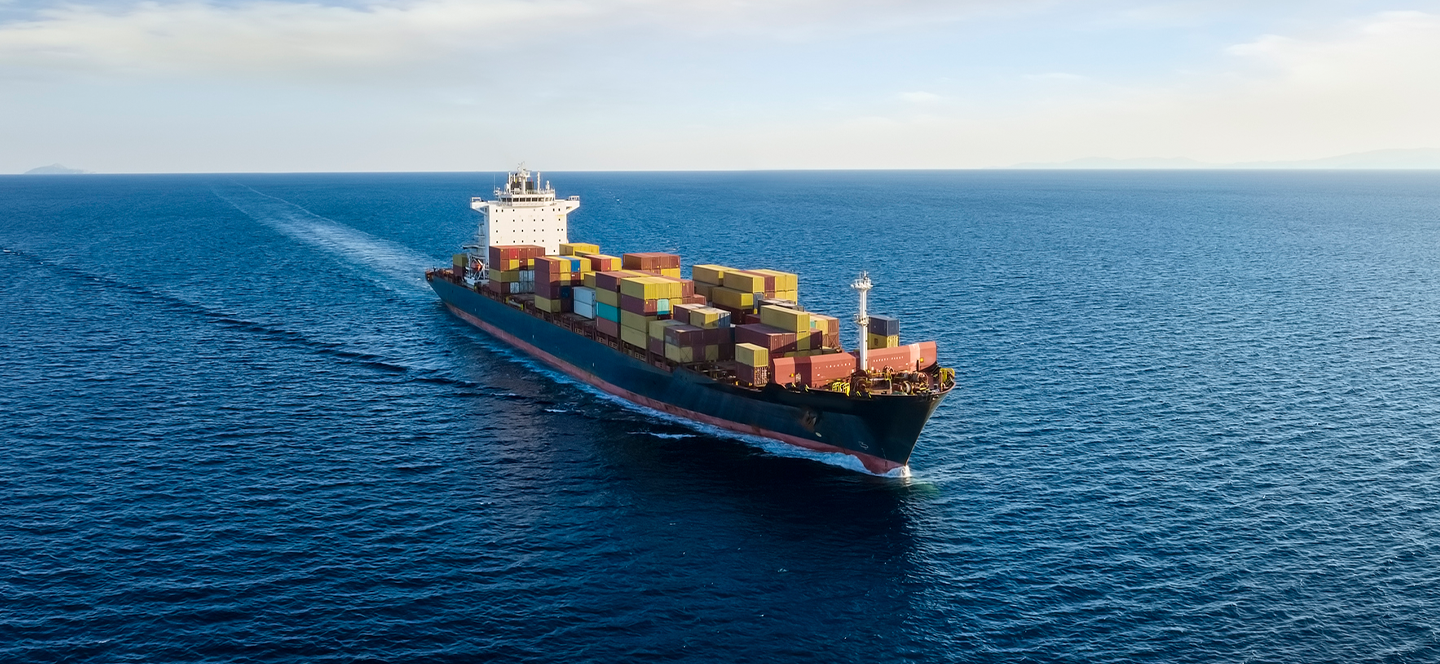
Carriers
<p>Providing data-led planning and coordination of trucking, ocean freight, air freight, and railway freight operations.</p>
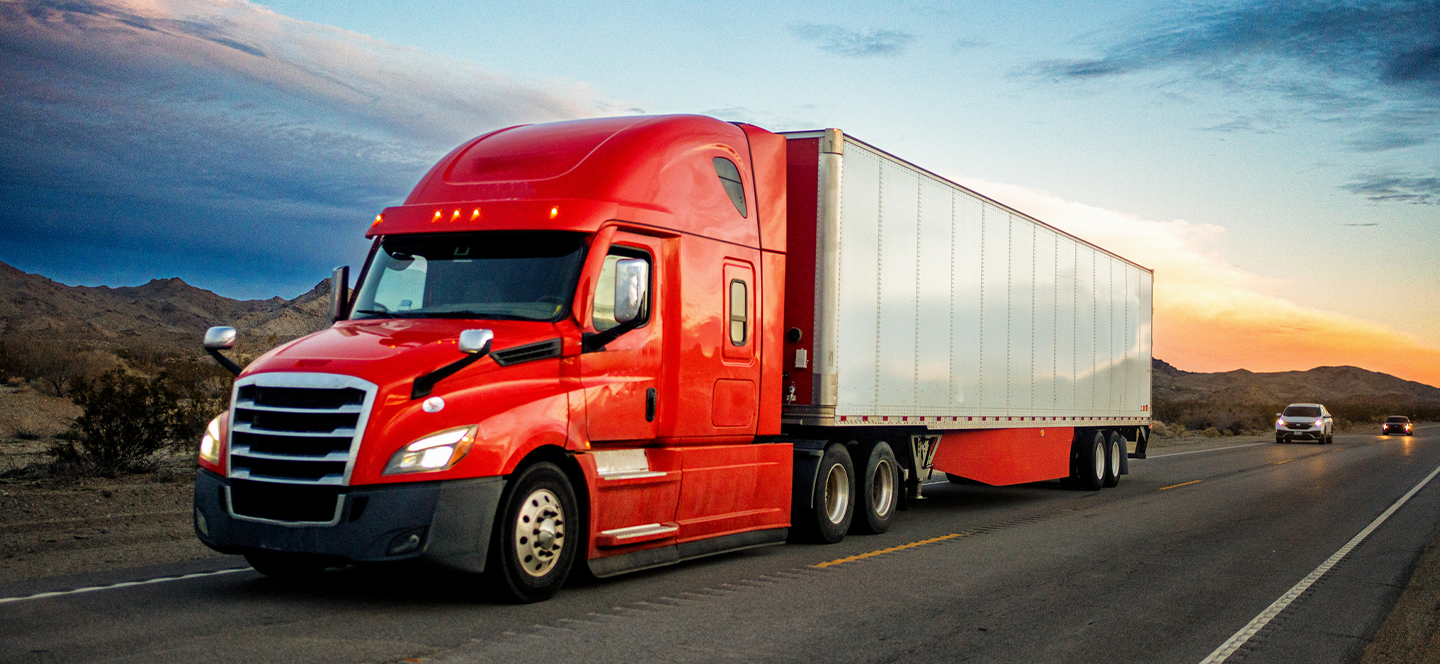
Logistics providers
<p>Transforming systems and processes for digital efficiency within third-party logistics, freight brokers, freight-forwarding organizations.</p>