An AI-Powered Intelligent Document Processing Solution driving exceptional value for clients!
Researches show that over 80% of data in enterprises are unstructured. There is immense value hidden under piles of unstructured content that remains unharnessed. Processes around extracting information from unstructured data is heavily manual, time consuming and error prone. Hence, companies are grappling with challenges of generating meaningful information from vast volumes of unstructured data.
EXL XTRAKTO.AI™ is Intelligent Document Processing solution that converts unstructured content into structured data with the power of AI and cloud technologies. EXL XTRAKTO.AI™ is an enterprise IDP solution which is template agnostic and can be scaled across multiple use cases. It comes with minimal technical debt for our clients and our commitment towards continuous customer and solution support. With thin human-in-the-loop layer, we ensure operationalization of AI models leading to end-to-end value generation.
With EXL XTRAKTO.AI™, our clients achieve highly accurate information at significantly high speed and lower cost. This results in improved process efficiencies, new data assets and insights for competitive advantage and market growth.
Why do operations need an intelligent document processing solution?
Manual data extraction processes in operations , typically grapple with the following challenges:
- Extensive variety and heterogeneity of content in terms of file formats (emails, PDFs, Excel etc.), document formats (structured vs unstructured) and print format (print, handwritten and mixed)
- Tedious repetitive steps that require capturing significant quantity of data points from vast volumes of documents. This involves time to search and time to punch the data on application.
- Inherent operational inefficiencies owing to:
- Manual referencing to entries to populate data into system
- Multiple screen toggles and clicks to update entries, leading to considerably high AHT and cost inefficiencies
- Manual allocation of cases leading to an inefficient inventory management process
- System latency
- Accuracy slips due to:
- Errors being committed due to incorrect procedural validations
- Error in typing (if documents are images)
- Errors due to human fatigue
- Unplanned volume fluctuations leading lower AHT expectations
What we do
EXL demonstrates significant breadth of proprietary AI/NLP models, frameworks and toolkit. EXL XTRAKTO.AI™ brings AI capabilities like advanced NLP and machine learning to accelerate document processing to meet business objectives for our clients. We believe that our framework is differentiated in four key dimensions:
Domain expertise
NLP modules incorporate vast domain expertise and experience (e.g. Medical ontology developed by our underwriting & claims SMEs for insurance use cases). We ensure output from our solution integrates seamlessly with operations workflow allowing human reps to manage exceptions and generate highly accurate data.
Advanced NLP capabilities
Proprietary stack of pre-built AI/NLP models and accelerator modules. These are developed by our team of talented and experienced data science professionals. These modules are quickly configured on our IDP platform as per use case requirements. Our stack speeds up AI model integration, ensures scalability and economies of scale across multiple use cases.
Customization
Every use case has its own nuance and uniqueness. ‘One size fits all’ approach can not be applied across the board for AI solutions. EXL XTRAKTO.AI™ is highly customizable as per business and technology requirements. IDP platform itself is shared across multiple use cases and AI models hosted are highly configurable as per use case at hand. We ensure solution is specialized for you.
Flexible deployment with modular components
We are flexible with our deployment options. EXL XTRAKTO.AI™ can be deployed for you on our secure cloud environment or it can also be deployed in your environment as per requirements. EXL XTRAKTO.AI™ is a modular solution hence its components can be customized and tuned as per your process and data requirements. Customization include AI models, integrations, Validation UI etc.
How is EXL XTRAKTO.AI™ different
AI/NLP modules have the following key features:
- Built using Deep Learning models such as BERT, spaCy and ML techniques (such as SVM)
- Capable of extracting text from unstructured and semi-structured documents
- Ability to handle a wide variety of document formats such as EML, DOC, DOCX and PDF
Solution highlights
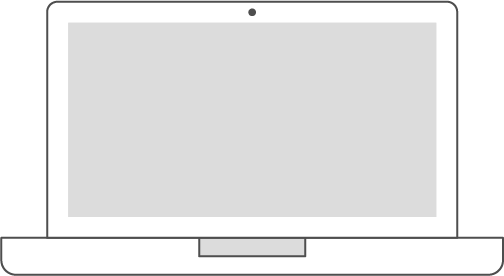
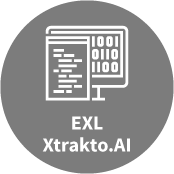
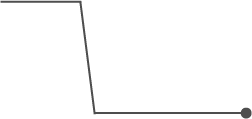
Integration with upstream systems, Ingestion and
pre-processing

Document classification and information extraction
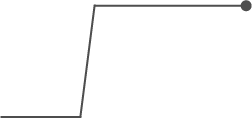
Post processing and Extracted data enrichment
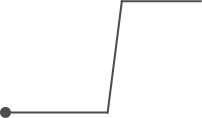
Human in the loop for validation of extracted data
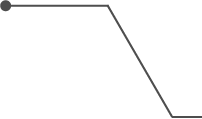
Output integration with downstream systems
- Integration with upstream systems, Ingestion and pre-processing
- Document classification and information extraction
- Post processing and Extracted data enrichment
- Human in the loop for validation of extracted data
- Output integration with downstream systems